Back in 2016 you might have read about a computer called AlphaGo that beat some of the best Go players in the world (I even wrote a blog post about it). At the end of 2020, DeepMind (the same company that developed AlphaGo), made news again. This time, their latest version of an artificial intelligence (AI)-driven program, AlphaFold2, has scored at the top in a biannual competition called Critical Assessment of protein Structure Prediction, or CASP. The goal of CASP is to use computer modeling to help predict how protein molecules fold, rather than having to do it all using laboratory experiments. The competition challenges teams of computational scientists to solve the folding structure of a protein at the same time as experimentalists are working on the same question. The team with the answer closest to the real-world experimental results wins the competition.
Not only did AlphaFold2 win, the scores for their predictions were so high that many news outlets, including the prestigious academic journal Nature, claimed that the protein folding problem is solved and “it will change everything.”1 Is it true? And if so – or even if almost so – what are the implications for sustainable nanotechnology specifically?

Let’s first briefly review the protein folding problem and try to appreciate why it is both important and challenging.
Proteins are the work horses of life. They carry out all the tasks essential to all living organisms – ranging from duplicating genetic materials (i.e., your DNA), to breaking down molecules from food to generate energy, to repairing damage to your cells from things like UV radiation from the sun. Conducting all these amazing functions requires proteins to adopt well-defined three-dimensional structures, which control how they interact with specific molecules and execute specific chemical modifications.

If you’ve ever put together any IKEA furniture, you know the importance of having parts of the right shape! Indeed, many human diseases are caused by mutations in the protein sequence (the order of amino acids that make up the protein) that alter the protein structure and therefore change its function. This is why, if we want to understand how proteins work and develop strategies to control what they do for medical purposes, an essential step is to figure out how a protein’s sequence determines its three-dimensional structure.

Why is this a challenging task?
The fundamental problem is that the number of possible three-dimensional structures (sometime referred to as “conformations”) that even a small protein can potentially fold into is enormous. The classical argument was made by molecular biologist Cyrus Levinthal – known as the Levinthal paradox – as early as 1969. Levinthal gave this example to explain the mind-boggling number of possible shapes a protein can fold into: Consider a modest-sized protein that consists of 100 amino acids. If each amino acid can adopt 10 possible conformations, and if we regard all the amino acids as being independent from one another, then the protein can potentially adopt 10100 different conformations! (Yes, that’s a googol.)
It is reasonable to assume that the shape that the protein actually forms will be the one with the most favorable energy, which means the shape in which all the chemical groups of the protein interact with each other in the best way. It’s kind of like how there are just a couple ways for a person to comfortably sit in a chair – other shapes might be possible, but they would not be “favorable”!
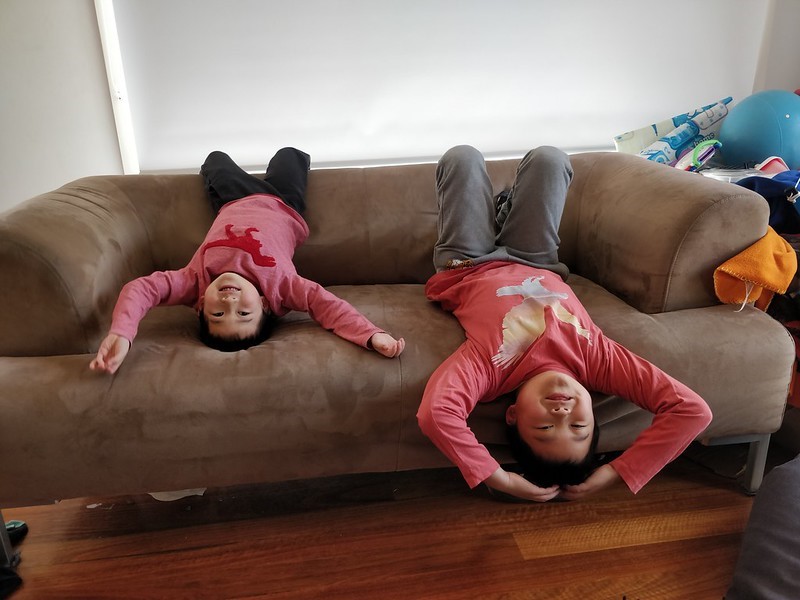
So for anyone trying to predict the shape of a protein by computer, the task is to identify the most stable conformation out of 10100 possible ones. To make things worse, many of these conformations are likely very close in energy, so the model has to be sufficiently accurate to identify the correct one among this astronomically large number of possibilities. This is much worse than finding a needle in haystack; considering that a haystack weighs typically 103 pounds, it is very unlikely that it contains even 109 pieces of hay! In fact, the current estimate for the number of atoms in the universe is only 1080!
So how can we go about tackling such a huge problem? There would be no way to just check every possible conformation one by one. Even if your program could test 100 per second, it would take about 32,749,526, 000,000,000,000,000,000,000,000,000,000,000,000,000,000,000,000,000,000,000,000,000,000,000,000,000,000,000,000,000 YEARS. So, you have to focus on at least two fundamental challenges:
- Efficiently sampling a huge number of possible conformations, either by looking at them in big batches or smartly reducing the number of conformations that you need to evaluate
- Properly ranking the likelihood that a particular conformation is the correct answer
The significance and intellectual challenge of the protein folding problem has attracted a large number of researchers from diverse fields that include physics, chemistry, structural biology and bioinformatics. The biannual CASP competition was founded in 1994 to help evaluate progress in the field. Participants are given a set of sequences and have a few weeks to submit their predictions; the predictions are then evaluated by comparing to experimental structures solved in the lab using X-ray crystallography, Nuclear Magnetic Resonance (NMR), or more recently, Electron Microscopy (EM). One particular metric to gauge how accurate the predictions are is the Global Distance Test – Total Score (GDT-TS), which measures the percentage of accurately-modeled amino acids in the predicted structure compared to the experimental one. Up until 2016 (CASP12), GDT-TS of about 40% was the limit for the prediction of difficult cases, which again highlights the challenge of the folding problem.

Even though it is very difficult, this is a challenge worth overcoming because experimental solutions of protein structures are often time-consuming and expensive, especially for large proteins, protein complexes and proteins that function in the cell membranes. Moreover, being able to predict accurate protein structures computationally also enables us to design new proteins of the right shape to realize new functions.
A multitude of strategies have been applied to attack the protein folding problem. Some approaches are “bottom-up” in nature, which evaluate the “quality” of each possible conformation based entirely on researchers estimating intermolecular interactions from their knowledge of fundamental physical and chemical principles. This approach is extremely challenging because small errors in the estimated energetics can accumulate and lead to incorrect prediction of low-energy structures.
Other approaches build on information available from known structures of proteins as well as known patterns in protein sequences. For example, if the protein of interest is very similar in sequence to another protein with a known structure, chances are they are also similar in three-dimensional conformation, so modelers can use the known structure as a “template” to guide the structural modeling process. Moreover, if particular features called amino acid identities on two sites within a group of related proteins are observed to change in a correlated way (i.e., they “co-evolve” in sequence), chances are those two sites are located close to each other in the three-dimensional structure. When this happens, it means that particular conformation is more favorable, giving researchers a clue that it is more likely to be accurate than other possibilities.
Thanks to the development of structural biology and sequencing technology, the amount of structural and sequence information available has been expanding at a remarkable pace. For example, the protein data bank (an open access archive of 3D structure data for biological molecules) now contains more than 170,000 entries, and the number of structures released annually has been at >11,000 for the past five years. Similarly, from 1982 to the present, the number of bases in GenBank (a genetic sequence databank) has doubled approximately every 18 months, and as of Feb 2021, it contains more than 260 million sequences, comprising more than 776 billion nucleotides. What does this have to do with protein folding? All of this available data means that a computational approach to the problem that takes advantage of the enormous structural and sequence information available has a good chance of succeeding. The challenge has become how to best integrate insights from both “bottom-up” models and “structural/sequence-informatics” approaches to construct structural candidates and rank them efficiently and accurately. Indeed, many of the groups who’ve done well in previous years’ CASP competitions have combined structural/sequence-informatics approaches with energy calculations motivated by physical models.
Why is AlphaFold2 considered “revolutionary”?
A research team from DeepMind joined CASP13 (the 13th competition) in 2018 with AlphaFold, a program based on a “deep neural network.” The depth of the neural network refers to the number of parameters in the model, which was about 21 million, and the model was trained based on a large amount of sequence and structural information for 29,000 known proteins. Although AlphaFold won CASP13, their GDS-TS for the most difficult targets was only 58.9, which was impressive but not that much better than the scores for the runner-up teams, which were around 52.
In 2020, DeepMind made the leap in CASP14 with AlphaFold2, which accomplished a median GDS-TS of 92.4. This means that the prediction had more than 92% of the amino acids in the protein in the correct conformation! This is the first time a computer model in the competition has reached a level of accuracy comparable to that of experimental techniques such as X-ray crystallography.

Even for a set of targets classified as the most difficult, the median score for AlphaFold2 was 87. Compared to the best score that hovered around 40 merely 4-5 years ago, this was indeed tremendous progress, and no wonder led to much excitement in the press. While DeepMind still has not officially released the algorithmic details of AlphaFold2, even experts in the field believe that revolutionary steps were taken to make such impressive improvement in prediction possible. Many people, myself included, eagerly await the official publication from DeepMind, since it is likely that their developments can find applications in other AI based problems related to proteins.
So what remains to be done?
It is important to point out that GDS-TS accuracy measurement is based only on the positions of certain atoms in the protein. A detailed description of the three-dimensional structure of a protein includes the positions of the amino acid sidechains as well. While John Moult (chair of the CASP competition) has stated that the side chain positions for the AlphaFold2’s predictions are “really really extraordinary,”4 the ability to “predict the shape of proteins to within the width of an atom” is far from being achieved. Perhaps most importantly, protein function requires a dynamic rather than a static structure. In other words, for proteins to properly function, they move through an ensemble of different shapes – they can “breathe” to allow them to stick to other molecules or release products that they make.
So, to truly revolutionize how we understand the relationship between sequence, structure, and function in proteins, we need to predict the relevant structural ensemble for a protein AND how it shifts in response to the interaction with other small molecules and biomolecules. Martin Karplus, the winner of the 2013 Nobel Prize in Chemistry, once described the relationship between static structures and dynamics of biomolecules in his memoir: “[Claude Poyart] likened the X-ray structures of proteins to a tree in winter, beautiful in its stark outline but lifeless in appearance. (Molecular) dynamics gives life to this structure by clothing the branches with leaves that flutter because of the thermal winds.”5 Literally and figuratively speaking, dynamics is essential to life.
How does the impressive accomplishment of AlphaFold2 impact research in the area of sustainable nanotechnology?
One big goal of the NSF Center for Sustainable Nanotechnology is to study how nanomaterials interact with the environment. “The environment” is obviously a big concept, but our research especially focuses on biomolecules, including proteins! It is essential to understand how nanomaterials interact with biomolecules and how such interactions potentially transform both of them structurally and chemically. Having an efficient approach to generate structural models for the relevant proteins is an important step towards achieving this goal, although achieving a microscopic understanding would require us to figure out how the protein structure changes due to nano/bio interactions. Based on that, we could develop models to predict the biological impact of nanomaterials. Looking further into the future, the ability to precisely predict protein structure could help us design new proteins with desirable structure, assembly and even catalytic properties for the purpose of controlling nano/bio interactions. Because of this potential, I anticipate that the AlphaFold2 technology will have both major short- and long-term impacts on sustainable nanotechnology research.
REFERENCES
- Callaway, E. ‘It will change everything’: DeepMind’s AI makes gigantic leap in solving protein structures. Nature News, Nov 2020. www.nature.com/articles/d41586-020-03348-4
- Mulnaes, D. et al. TopModel: Template-Based Protein Structure Prediction at Low Sequence Identity Using Top-Down Consensus and Deep Neural Networks. Journal of Chemical Theory and Computation : JCTC. 2020, 16(3), 1953-967. doi: 10.1021/acs.jctc.9b00825
- Hou, J. et al. Protein tertiary structure modeling driven by deep learning and contact distance prediction in CASP13. Proteins, Structure, Function, and Bioinformatics. 2019, 87(12), 1165-1178. doi: 10.1002/prot.25697
- Moult, J. CASP 14 Critical Assessment of Structure Prediction– Advancing solutions to the Protein Folding Problem. National Institute of General Medical Sciences. 2020. Presentation slide 19. Video from 22:46.
- Karplus, Martin. Spinach on the ceiling: A theoretical chemist’s return to biology. Annual Review of Biophysics and Biomolecular Structure. 2006, 35(1), 1-47. doi: 10.1146/annurev.biophys.33.110502.133350